As artificial intelligence (AI) continues to evolve and become integral to various industries, ensuring its security has emerged as a critical concern. This article delves into the challenges and strategies for safeguarding AI, focusing on how organizations can protect their AI systems effectively.
Understanding the Landscape of AI Security
The Growing Importance of AI Security
AI has become a cornerstone technology for numerous sectors, offering transformative potential but also introducing new security vulnerabilities. Recent studies show that 98% of IT leaders acknowledge AI’s significance for their business operations. However, 77% of these leaders reported security incidents related to AI within the past year, underlining the pressing need for robust security measures.
Examples of potential security threats include:
- Data Poisoning: Malicious actors can manipulate training data to alter AI model behavior, leading to biased or incorrect predictions. Microsoft’s chatbot Tay is a notable example, where manipulated user inputs turned the AI into a source of harmful content within 16 hours of its launch.
- Model Evasion: Attackers use adversarial inputs to mislead AI models. These attacks can bypass spam filters, malware detection systems, and even self-driving car systems by subtly altering inputs to cause misclassifications.
- Model Theft: Attackers target AI models to replicate them or extract sensitive data. Techniques such as extraction attacks, inversion attacks, and membership inference attacks compromise data privacy and intellectual property.
- Prompt Injection: Attackers use specially crafted prompts to bypass AI model restrictions, enabling them to perform unauthorized actions. This highlights the need for better security filters and guardrails.
- Code Injection: Some AI models can execute user-provided code, posing risks of arbitrary code execution. Ensuring that AI applications do not run unverified code is crucial for security.
- Supply Chain Attacks: Compromising trusted vendors to introduce malicious components into AI products can lead to widespread security breaches. Organizations must adapt their security controls to address these vulnerabilities.
Addressing AI Security Risks
Addressing AI security risks requires a multifaceted approach to protect against various threats. Data poisoning attacks occur during model training, where malicious data can compromise the reliability of AI systems, especially in continuous learning environments. To mitigate this risk, organizations should validate and monitor their training data sources and implement processes to detect and prevent data manipulation.
Model evasion attacks target deployed AI models by attempting to understand and bypass their predictions, posing threats to systems such as self-driving cars or facial recognition. To counter these attacks, adversarial training techniques can improve model robustness, and continuous monitoring of model outputs can help detect evasion attempts.
Model theft attacks, which involve replicating or extracting sensitive data from AI models, are another concern. Organizations can protect against these by using secure APIs, limiting access to model details, and employing techniques like differential privacy to safeguard training data.
Prompt injection and code injection attacks exploit weaknesses in generative AI models and code execution, potentially compromising systems. To mitigate these risks, strict guardrails and content filters should be established, and unverified code should be prevented from running through code review processes.
Finally, supply chain attacks pose a risk when trusted vendors are compromised, introducing malicious components. Organizations should conduct thorough security assessments of third-party vendors and implement tight supply chain security controls along with continuous monitoring to defend against such attacks.
Strategies for Strengthening AI Security
Despite the numerous security risks associated with AI, there are many tools and practices that can strengthen AI security, such as:
- Offensive and Defensive Tooling: Utilize tools like Metasploit, Cleverhands, IBM’s ART, and Microsoft’s Counterfeit for testing and improving AI security. These tools help identify vulnerabilities and strengthen defenses.
- Defensive Frameworks: Implement comprehensive security frameworks with practices, strategies, and recommendations for AI. Frameworks like MITRE Atlas provide valuable guidance for safeguarding AI systems.
- Regulations and Policies: Stay informed about global AI regulations and policies. Examples include Singapore’s Model AI Governance Framework, Canada’s Digital Charter Implementation Act, and the U.S. Blueprint for AI Bill of Rights. These frameworks guide ethical AI development and deployment.
- Collaboration and Communication: Foster collaboration between data scientists and cybersecurity teams. Ensuring alignment and effective communication is crucial for securing AI systems.
Predictions and Recommendations
As we move forward, it is essential to stay ahead of emerging threats, as well as improve communication amongst teams. As AI continues to evolve, we can expect to see more:
- Increase in Deep Fakes: Expect a rise in deep fake incidents, necessitating advanced detection and prevention measures.
- Collaborative Efforts: Data scientists and cybersecurity professionals will increasingly work together to address AI security challenges.
- Expanding AI Attack Surfaces: Organizations will need to use advanced tools and techniques to combat evolving threats.
To manage cyber risks effectively, organizations should regularly assess their security measures and ensure they are data-driven and unbiased. Implementing comprehensive security practices from the design phase through post-deployment is critical for safeguarding AI systems. By understanding the risks, implementing tight security measures, and fostering collaboration, organizations can protect their AI investments and ensure the safe deployment of this emerging technology.
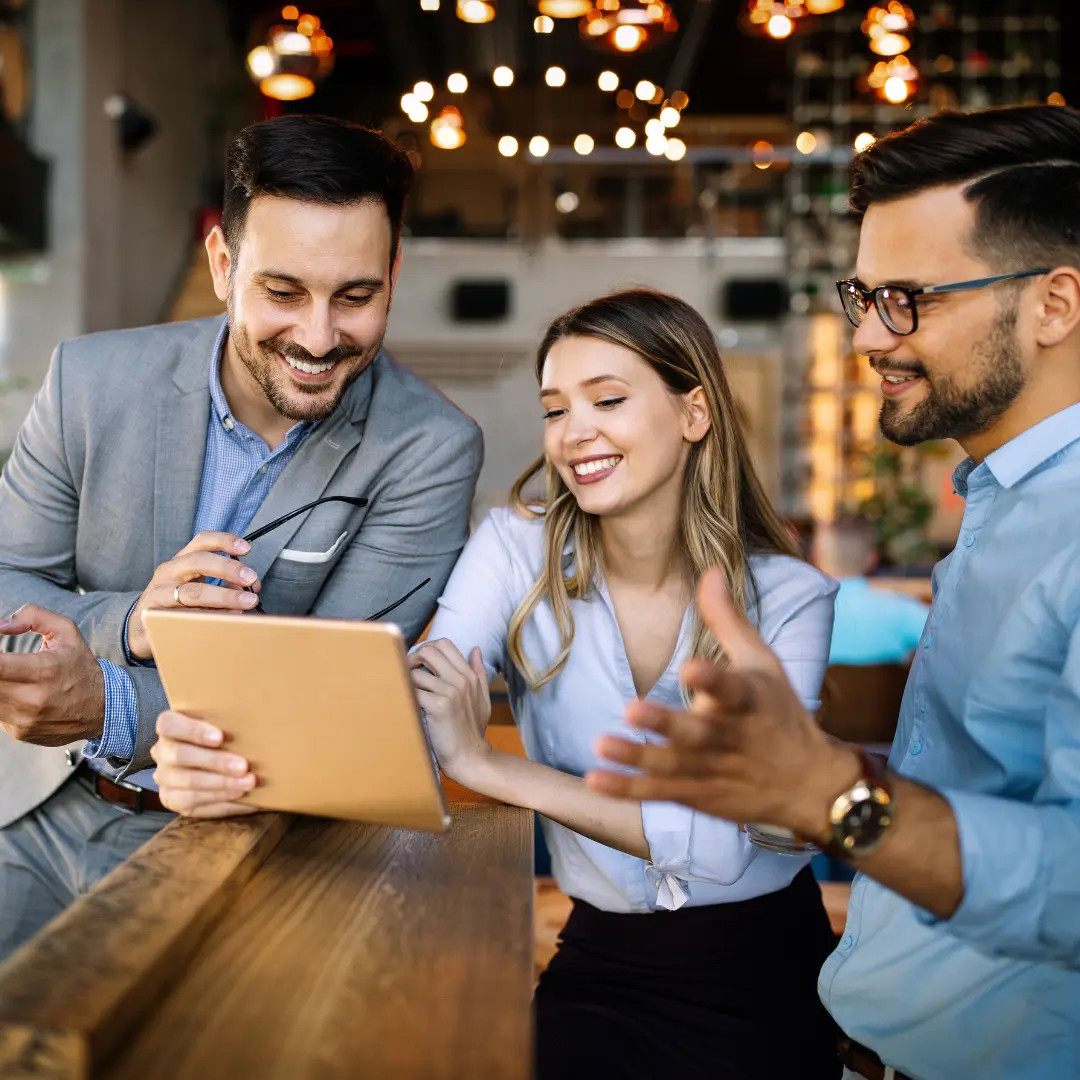
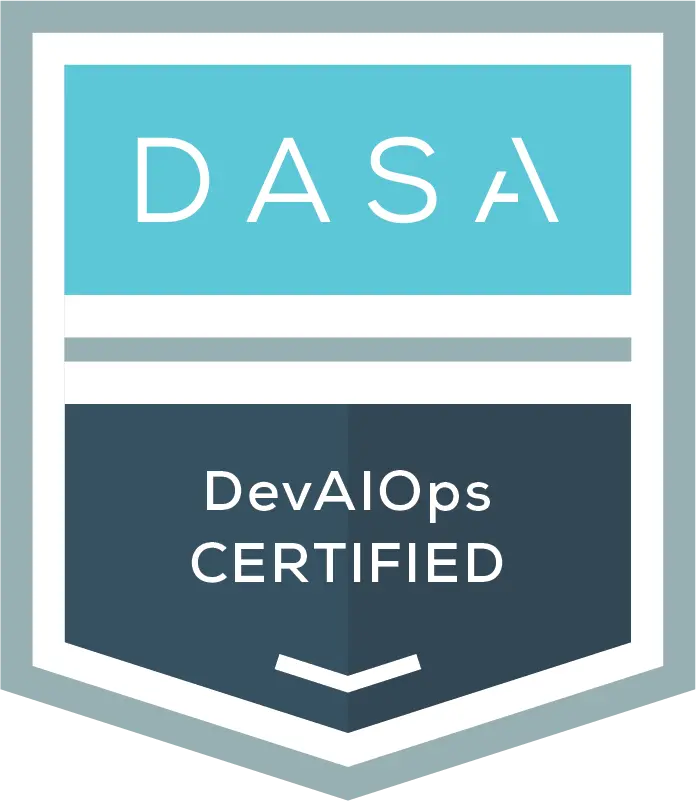
DASA DevAIOps
Adopt and adapt DevAIOps practices effectively, achieving faster time-to-market and time-to-value.